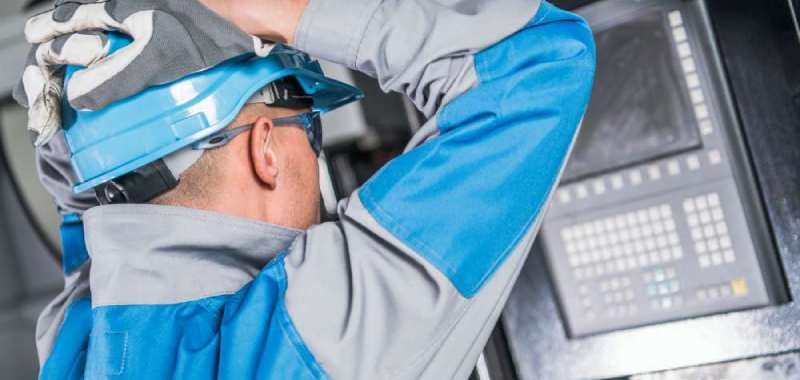
The 10 most common mistakes about “machine learning”
The Spanish Data Protection Agency (AEPD) and the European Data Protection Supervisor (EDPS) have published a new joint document in which they expose the 10 most common mistakes related to machine learning and provide an analysis of what the correct approach should be.
In this regard, the EDPS’s role is to ensure that, when processing personal data, EU institutions and bodies respect citizens’ right to privacy.
The aim of this paper is to elucidate the most common misconceptions surrounding machine learning systems, as well as to underline the importance of implementing these technologies in accordance with EU values, data protection principles and respect for the rights of individuals.
Before addressing these aspects of the aforementioned document, it is particularly important to go back in time, as the EU has highlighted the importance of Artificial Intelligence in the strategy for the digital transformation of the EU.
Machine learning is a specific branch of AI, applied to solving specific and limited problems, such as classification or prediction tasks. Unlike other types of AI that attempt to emulate human expertise (e.g. expert systems); the behavior of machine learning systems is not defined by a predetermined set of instructions.
The 10 most common errors:
1. Correlation implies causation.
In this sense, machine learning systems are very efficient at finding correlations, but lack the analytical capacity to go beyond that and establish a causal relationship.
2. When developing machine learning systems, the more data and the greater the variety the better.
Training machine learning systems requires large amounts of data, depending on the complexity of the task to be solved. However, using more training data in the development of machine learning models will not always improve system performance.
3.Machine learning needs completely error-free training data.
The performance of machine learning models depends, among other factors, on the quality of training, validation and test data. Therefore, these data sets must be able to define a real case in a sufficiently complete and accurate way.
4.The development of machine learning systems requires large data repositories or the sharing of data sets from different sources.
Clustering data and the machine learning system in a cloud computing infrastructure controlled by the machine learning system developer is a common solution to avoid performance constraints.
5.Machine learning models automatically improve over time.
A model that is implemented and no longer trained will no longer “learn” correlations from incoming data, no matter how much data is provided to it.
6.Automatic decisions made by machine learning algorithms cannot be explained.
Different degrees of detail may be needed in the explanation of the model, depending on the individuals and the context.
7.Transparency in machine learning violates intellectual priority and is not understood by the user.
When processing personal data using machine learning, data controllers should adequately inform data subjects about the possible impacts on their daily lives.
8.Machine learning systems are subject to less bias than humans themselves.
The aim of machine learning systems is to reflect the experience and knowledge provided by their creators.
9.Machine learning can accurately predict the future.
Machine learning systems take into account the data present in data sets and use it to extract projections of possible future outcomes.
10.Stakeholders are able to anticipate the possible outputs that machine learning systems can provide with their data.
Machine learning systems are excellent at finding correlations in data. They are able to identify patterns in personal data that go beyond those explicitly stated in the model development, and that might be unknown even to the individuals concerned (e.g., a predisposition to a disease). This potential raises several concerns from a data protection point of view.
Machine learning techniques are needed to improve the accuracy of predictive models. Depending on the nature of the business problem being addressed, there are different approaches based on the type and volume of data.

Letslaw es una firma de abogados internacionales especializada en el derecho de los negocios.